2025-05-06 19:22:00
www.variance.co
The Flattening Calibration Curve
The post-training process for LLMs can bias behavior for language models when they encounter content that violates their safety post-training guidelines. As mentioned by OpenAI’s GPT-4 system card, model calibration rarely survives post-training, resulting in models that are extremely confident even when they’re wrong.¹ For our use case, we often see this behavior with the side effect of biasing language model outputs towards violations, which can result in wasted review times for human reviewers in an LLM-powered content moderation system.
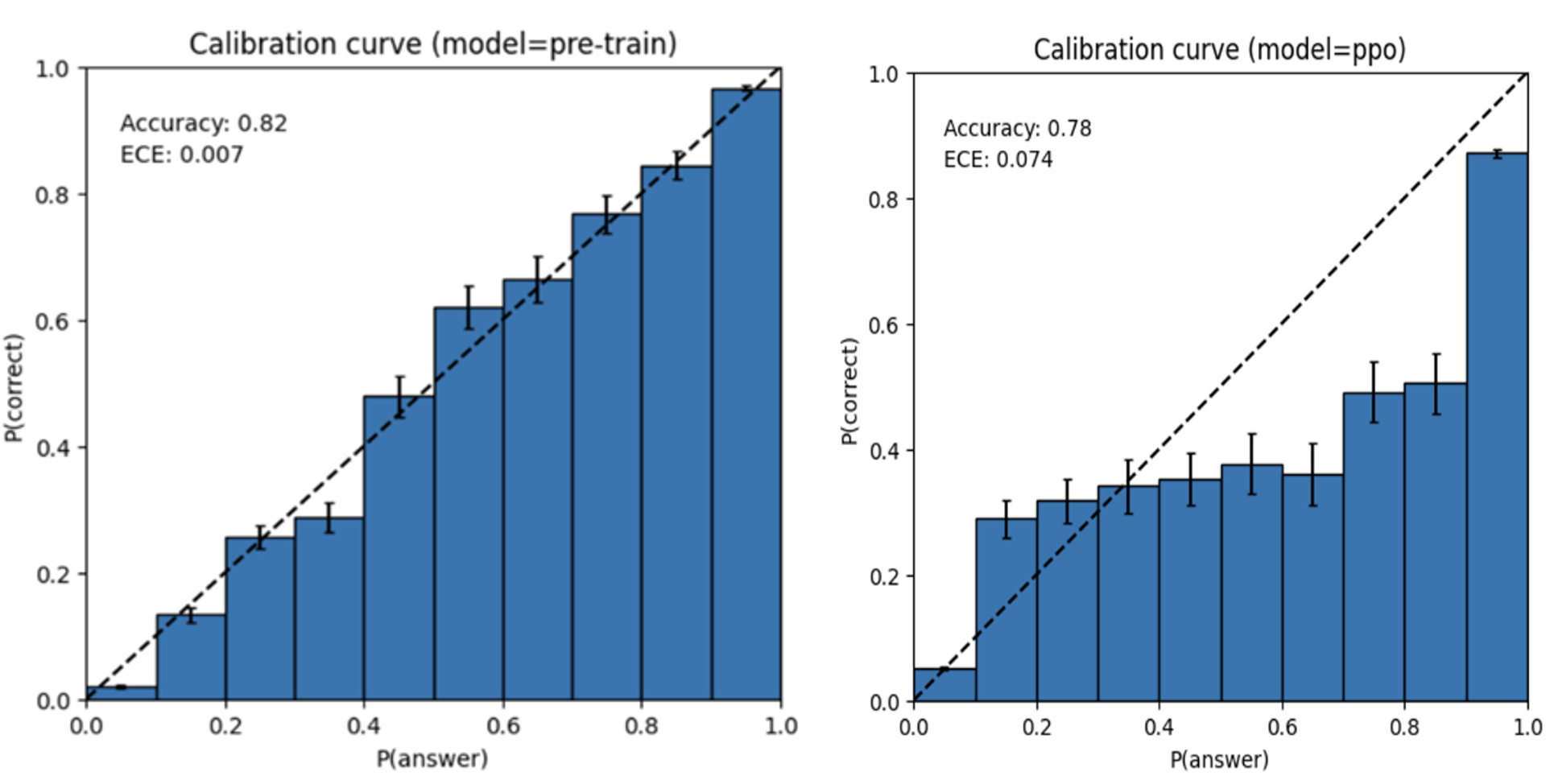
A Working Signal on GPT-4o
Take the below histogram of log probs sampled from a golden dataset of false positives against GPT-4o. We can see that almost all outputs have log p≈0 nats (probability ≈ 1) for outputting “true”, indicating a true violation in this dataset.
However, there are a few outliers in this dataset, almost all of which correspond to patterns of behavior we observed in our dataset when our model would stray away from formal grounded policy definitions, or hallucinations in content or policy violations.
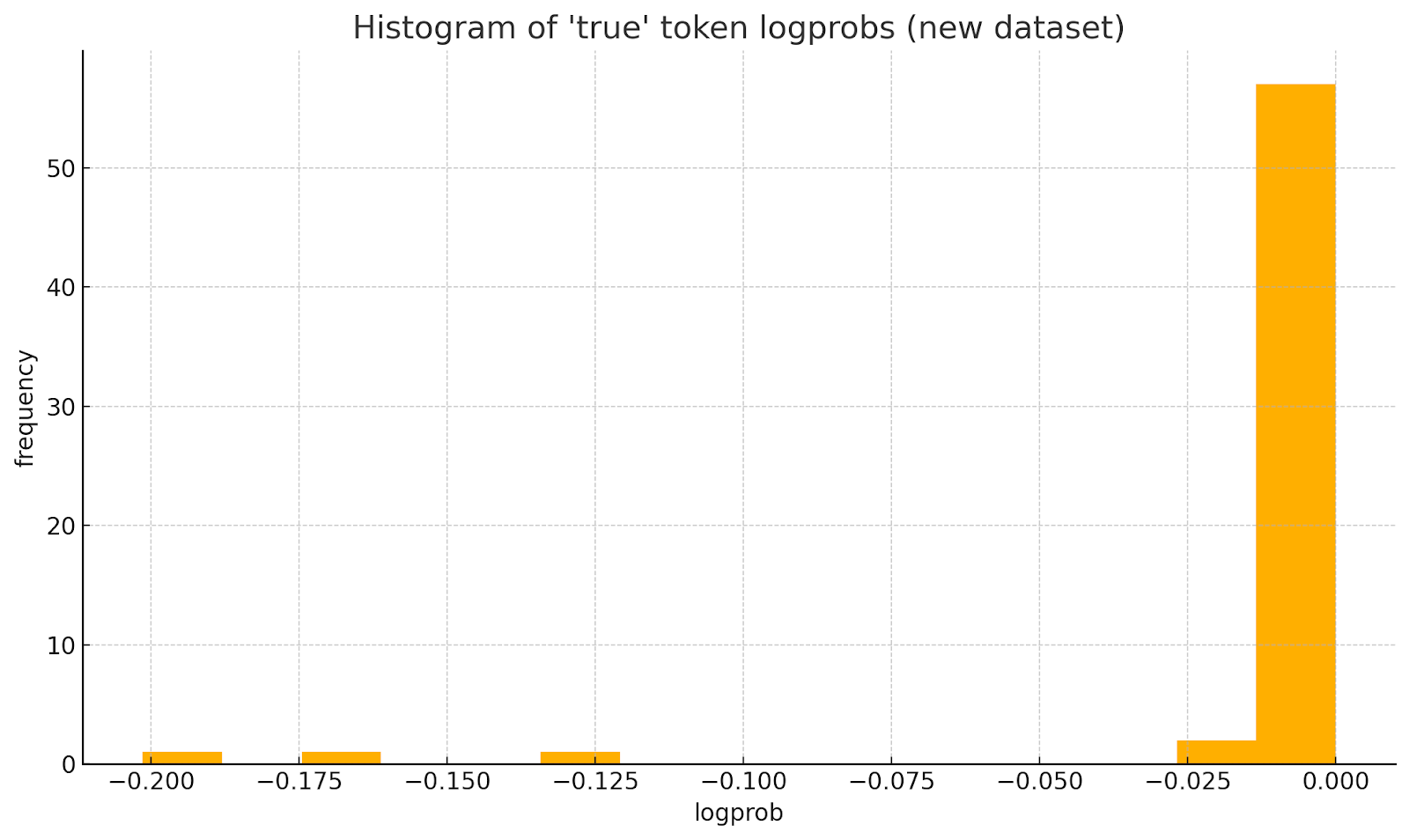
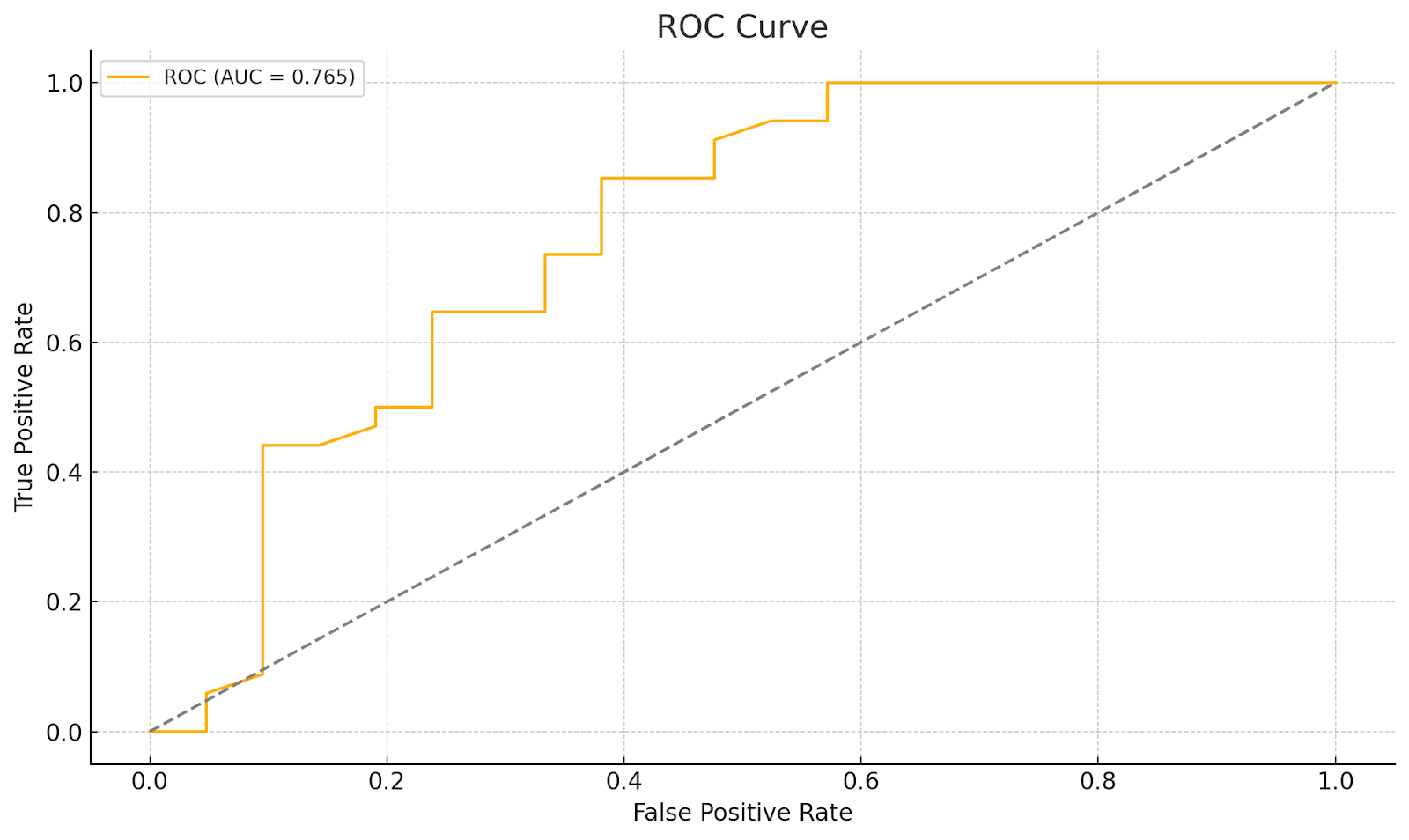
This results in a functional enough ROC curve that’s helpful for calibrating our model to ignore these outputs, and perform tasks like flagging the content for review or suppress the output as likely spurious.
The Upgrade That Vanished Uncertainty
However, what we found is that after switching to GPT-4.1-mini, this signal vanishes. Although we’re still able to measure log probs for other tokens in our structured outputs, each token was 100% confident that it should return true in this dataset, which completely destroyed our signal.
Why does a smaller sibling of the same model family erase so much information? It’s possible that due to the heavy distillation that occurs to train 4-1 mini for binary decisions (such as outputting a boolean field in a structured output), the dimension is collapsed entirely: the student is taught to emit the right answer and ignore entropy at all. This results in no usable confidence signal.
We tried several other approaches to recover the lost uncertainty signal, all unsuccessful:
- Entropy differential hypothesis: We measured entropy between content array vs. chain-of-thought mean, with the theory that hallucinated violations would be wordier/less confident. In practice, we were unable to find a signal here
- Span consistency check: We analyzed standard deviation of span log-probs, hoping for variation between true/false cases. In practice, both classes showed σ≈0.018 (identical).
- Perplexity analysis: We calculated token-level perplexity averages across all samples. In practice, we found similar metrics for every sample, safe or unsafe.
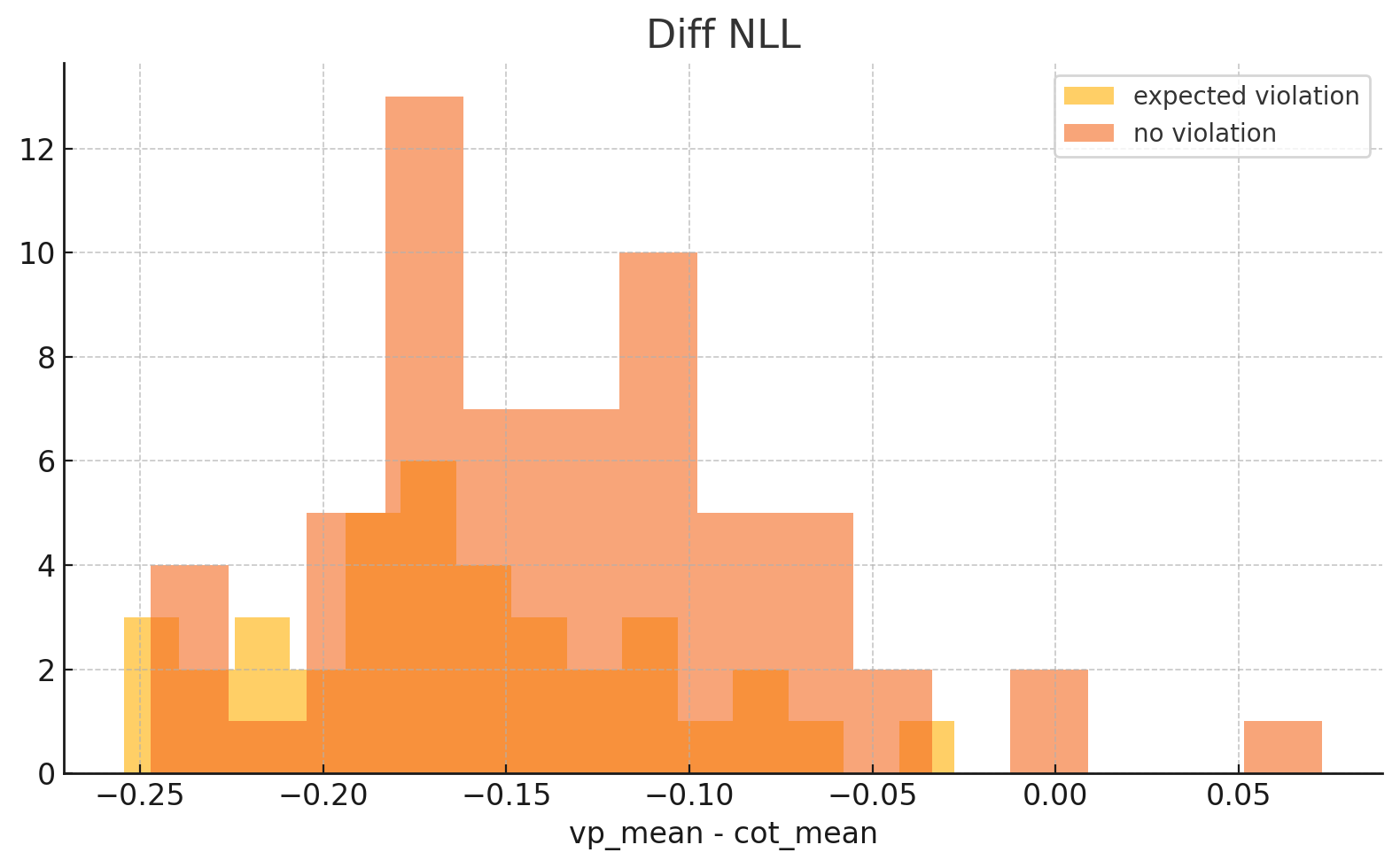
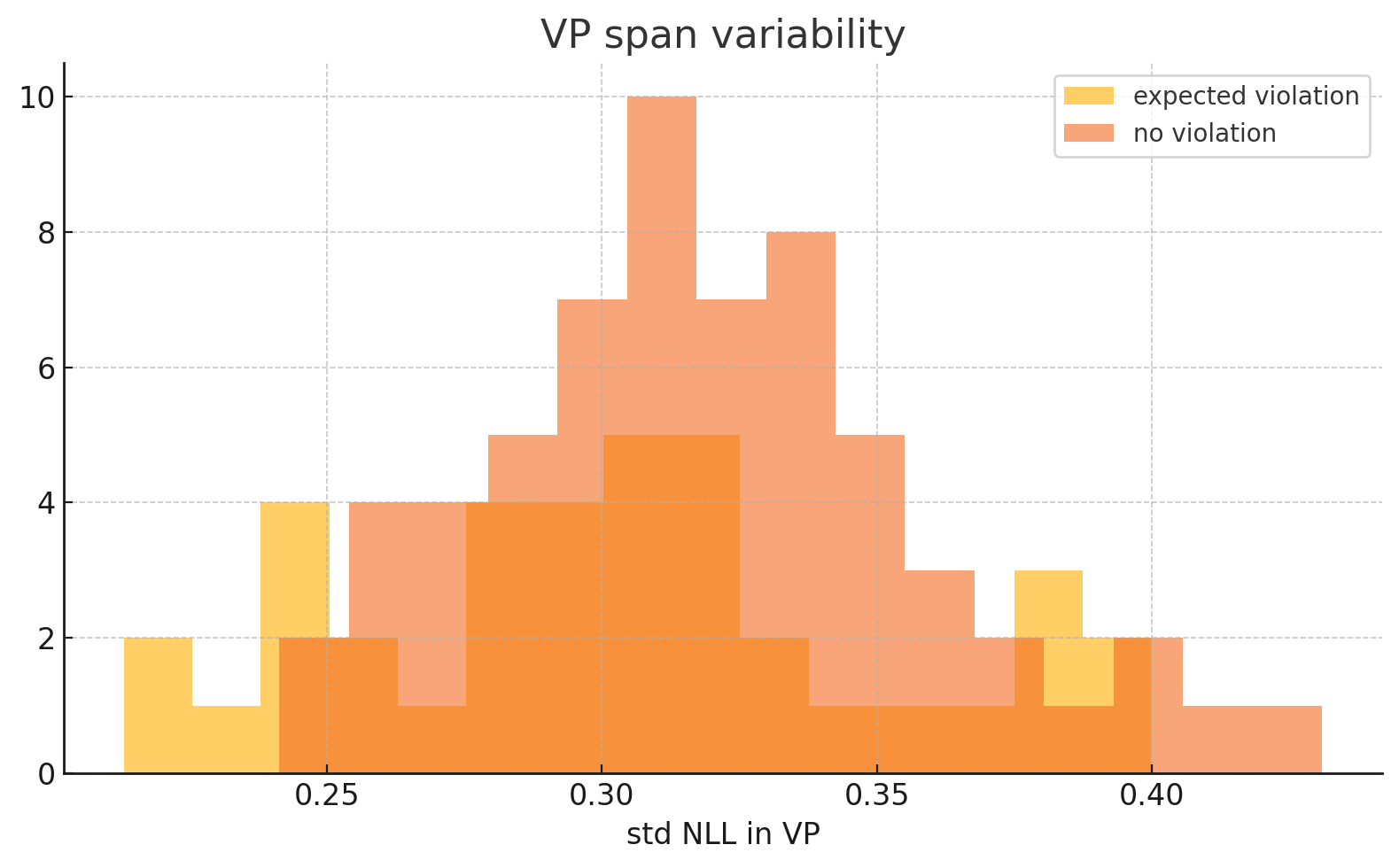

The net result is that we’ve lost our signal for hallucinations! All of these features rely on local entropy surviving RLHF, and we don’t have anywhere to look for these signals, requiring new heuristics for model upgrades to solve these failure cases, to re-introduce some uncertainty measures.
In response to this lost hallucination signal, we’ve implemented several alternative safeguards. These new methods, such as formally requiring policy explanations to be fully grounded in actual data/quotes, are powering new features in our product towards better explainability and policy iteration, but do show how there’s more to model upgrades than simply benchmark upgrades.
Our current approach relies on more explicit controls: requiring detailed explanations from the model for each policy violation, demanding specific policy citations to ground decisions, and implementing filtering systems to catch corrupted outputs when policies are hallucinated.
However, the closed-source nature of these models significantly limits our access to internal signals beyond log probabilities. As models continue to be further distilled for efficiency, even these limited signals are fading, creating a growing challenge for reliable uncertainty detection especially when working with closed-source models.
Alignment isn’t free
In our situation, the improvements to steerability and performance upgrades of 4.1 were worth it for customers and our internal workarounds were sufficient to actually increase precision with our latest release. A model upgrade is not merely a drop-in performance bump; it is a distributional shift that can invalidate an entire AI stack. Anyone shipping high-precision systems should log raw logits, tie heuristics to specific model versions, and invest in alternative product safeguards. Alignment makes models safer for users but simultaneously masks their own uncertainty from engineers; the burden of re-exposing that uncertainty falls on us.
1. *OpenAI GPT‑4 System Card*, § 6.2 “Calibration”: “We observe that RLHF improves helpfulness but can distort the model’s probability estimates; after alignment the model tends to be over‑confident on both correct and incorrect answers.
Keep your files stored safely and securely with the SanDisk 2TB Extreme Portable SSD. With over 69,505 ratings and an impressive 4.6 out of 5 stars, this product has been purchased over 8K+ times in the past month. At only $129.99, this Amazon’s Choice product is a must-have for secure file storage.
Help keep private content private with the included password protection featuring 256-bit AES hardware encryption. Order now for just $129.99 on Amazon!
Help Power Techcratic’s Future – Scan To Support
If Techcratic’s content and insights have helped you, consider giving back by supporting the platform with crypto. Every contribution makes a difference, whether it’s for high-quality content, server maintenance, or future updates. Techcratic is constantly evolving, and your support helps drive that progress.
As a solo operator who wears all the hats, creating content, managing the tech, and running the site, your support allows me to stay focused on delivering valuable resources. Your support keeps everything running smoothly and enables me to continue creating the content you love. I’m deeply grateful for your support, it truly means the world to me! Thank you!
BITCOIN bc1qlszw7elx2qahjwvaryh0tkgg8y68enw30gpvge Scan the QR code with your crypto wallet app |
DOGECOIN D64GwvvYQxFXYyan3oQCrmWfidf6T3JpBA Scan the QR code with your crypto wallet app |
ETHEREUM 0xe9BC980DF3d985730dA827996B43E4A62CCBAA7a Scan the QR code with your crypto wallet app |
Please read the Privacy and Security Disclaimer on how Techcratic handles your support.
Disclaimer: As an Amazon Associate, Techcratic may earn from qualifying purchases.